The crucial role of social media posts in tracking COVID-19 infection rates
已发布 26 八月, 2021
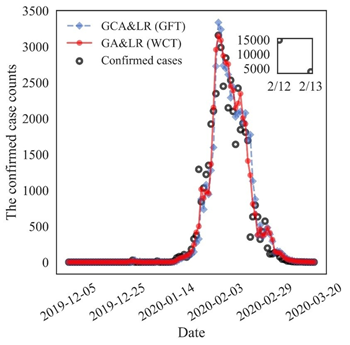
Monitoring and understanding the dynamics of the COVID-19 epidemic is critical as countries struggle to keep infections under control.
Out of necessity, incomplete non-medical data has been integrated into prediction models for the epidemic, but the accuracy and generalisability of the data are difficult to guarantee.
In a study published in the KeAi journal Data Science and Management, a group of researchers from China set out to assess the ability and applicability of using social media data to predict the development of COVID-19. Drawing on the existing Google Flu Trends (GFT) algorithm, they created a new, confirmed case prediction algorithm called Weibo COVID-19 Trends (WCT).
The research team was led by Xin Lu, a professor at the National University of Defense Technology’s College of Systems Engineering in China, who specializes in modelling the spread of epidemics. And according to Professor Lu: “The training model we developed effectively filters out mass online social media content. As a result, the correlation between our prediction and the real case data is as high as 0.98 the day before official figures are released, and 0.86 over an eight-day prediction period.”
To achieve those high scores, the team created a genetic algorithm that automatically constructs a keyword set for filtering COVID-19 related posts. They applied it to the Chinese microblogging website, Sina Weibo, looking at posts made by users in Wuhan, China, where the virus first emerged. The resulting dataset was then combined with the number of historical COVID-19 case counts.
Professor Lu explains: “We found that the relative frequency of certain keywords in the Weibo post dataset was very similar to the trend we were seeing in the number of new, confirmed cases of COVID-19. The method consistently outperformed the maximum average test score in the training set. It also improved on the results obtained by the Google Flu Trends (GFT) algorithm, largely because it addressed GFT’s shortcoming of over-estimating the epidemic peak value. We found that WCT is much more stable than GFT – predictions do not fluctuate when the parameters change, e.g., the duration of training data.”
The authors believe the study offers a highly-adaptive approach for feature engineering of third-party data in epidemic prediction and provides useful insights for the early prediction of newly-emerging infectious diseases at a stage when most epidemiological characteristics typically remain unknown.
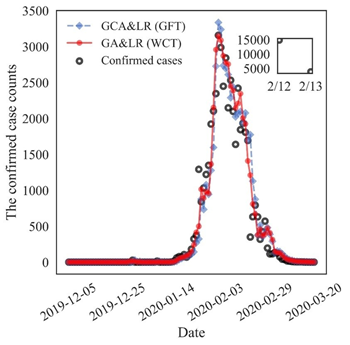
Contact the corresponding author: Professor Xin Lu, xin_lyu@sina.com