Artificial General Intelligence for Radiation Oncology
Published 02 January, 2024
An estimated 600,000 people in the United States succumb to cancer every year. Beyond surgery, chemotherapy, and immunotherapy, radiotherapy has shown to be a standard and effective treatment option for nearly 50-70 percent of cancer patients.
Radiotherapy treatment involves six basic stages: initial consultation, simulation, treatment planning, treatment delivery, treatment verification, and patient follow-up. With the emergence of Artificial intelligence (AI), radiotherapy is undergoing substantial transformation. AI achieves human-level accuracy in auto-segmentation, tumor staging, image registration, automatic treatment planning, quality assurance, and outcomes prediction, which greatly improves the accuracy, precision, and efficiency of radiation therapy.
According to a recent review published in the KeAi journal Meta-Radiology, pioneering AGI models, such as GPT-4 and PaLM 2, along with large vision models (LVMs) like the Segment Anything Model (SAM), are capable of processing extensive texts and imaging data, respectively. AGI, distinguished by its advanced capabilities in few-shot and zero-shot learning, offers a pathway toward developing highly robust and generalizable AI models. These models are imperative for seamless and effective integration into the various facets of radiation oncology.
They found that the fusion of vision data with LLMs also creates powerful multimodal models that elucidate nuanced clinical patterns. Together, AGI has the potential to catalyze a shift towards data-driven, personalized radiation therapy. The authors stressed that these models should complement human expertise and care.
The findings provide an overview of how AGI can transform radiation oncology to elevate the standard of patient care, with the key insight being AGI’s ability to exploit multimodal clinical data at scale. In addition, the researchers explore the future directions of AGI in radiation oncology and discuss potential developments and bottlenecks in this field. These advancements in clinical applications will further enhance the effectiveness of radiation therapy, bringing more positive outcomes for cancer patients.
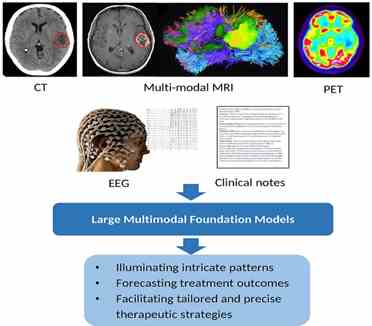
Contact author name, affiliation, email address: Wei Liu, Department of Radiation Oncology, Mayo Clinic, Liu.Wei@mayo.edu
Conflict of interest: The authors declare that they have no known competing financial interests or personal relationships that could have appeared to influence the work reported in this paper. The author is an Editorial Board Member for Meta-Radiology and was not involved in the editorial review or the decision to publish this article.
See the article: Liu C, Liu Z, Holmes J, Zhang L, Zhang L, Ding Y, et al. Artificial General Intelligence for Radiation Oncology. Meta-Radiology. 2023:100045. https://doi.org/10.1016/j.metrad.2023.100045