Breakthrough in Predicting Cancer Recurrence: AI Model Enhances Prognostic Accuracy with Multi-Omics Integration
Published 25 April, 2025
Predicting tumor recurrence at the molecular level emerges as a critical challenge, especially for patients with complex multi-omics profiles. Traditional prognostic models relying on single biomarkers fail to sufficiently capture the interactions between genomic, epigenetic and transcriptomic drivers.
In particular, multi-omics integration face two key obstacles: the reliance on empirical feature selection limiting cross-cancer applicability and the enormous computational complexity in interpreting biological pathways. To that end, in a study published in Fundamental Research by a team from Central South University and Guangxi University, led by Prof. Jianxin Wang and Dr. Wei Lan, the researchers proposed a novel framework that enables precise and interpretable recurrence risk prediction.
"When analyzing multi-omics data from cancers such as bladder and gastric cancer, traditional machine learning models struggle to capture pathway-level interactions," explains Lan. “Our new framework, MULGONET, overcomes this limitation through two key advances.”
The MULGONET framework
- Gene ontology guided architecture by building a hierarchical network based on more than 11,000 Gene Ontology (go) terms, MULGONET automatically links genes (such as Cdk6) to biological processes (such as "cell cycle regulation" go:0051726), eliminating the need for manual feature selection. This enabled the application to trans-cancer, with AUPR scores of 0.774 (bladder cancer), 0.873 (pancreatic cancer) and 0.702 (gastric cancer).
- Effective multi-omics fusion The model's attention-based fusion mechanism processes 3D omics data (gene × omics layer × GO term) in less than 2 hours on standard hardware, while existing tools require more than 8 hours.
"Our framework can not only predict recurrence, but also identify driver pathways," says Lan. "For example, in pancreatic cancer, a high attributable score of Wnt5a in the" G protein-coupled receptor signaling pathway "(go:0007186) is associated with early recurrence."
The team has opened its framework to accelerate community-driven applications. "We hope that MULGONET can stimulate new research on the interpretability of multi-omics," adds Wang.
The study, published in KeAi’s Fundamental Research, directly supports precision oncology by revealing viable targets of Rock1 in metastasis.
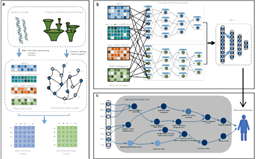
Contact author: Jianxin Wang, National Engineering Laboratory of Medical Big Data Application Technology, Central South University, Hunan 410083, China, jxwang@csu.edu.cn
Funder: This paper was supported by the National Natural Science Foundation of China (U24A20256, 62472128); the Natural Science Foundation of Guangxi (2024GXNSFFA010006); the Guangxi Bagui Youth Talent Program; the Project of Guangxi Key Laboratory of Eye Health (GXYJK-202407); the Project of Guangxi Health Commission Eye and Related Diseases Artificial Intelligence Screen Technology Key Laboratory (GXYAI-202402).
Conflict of interest: The authors declare that they have no conflicts of interest in this work.
See the article: Wei Lan, Zhentao Tang, Haibo Liao et al., MULGONET: An interpretable neural network framework to integrate multi-omics data for cancer recurrence prediction and biomarker discovery. Fundamental Research, https://doi.org/10.1016/j.fmre.2025.01.004