Estimating reward & risk with machine learning improves portfolio performance by up to 28%
Published 23 February, 2022
Machine learning (ML) in asset management is an increasingly popular domain. Many studies and asset managers focus on forecasting returns with machine learning models to form investment portfolios that beat the market.
However, they often rely on simple and static estimates of market volatility, in other words, the speed with which stock prices rise and fall over time. This results in portfolio weights that are less than optimal.
In a study published in KeAi’s The Journal of Finance and Data Science, Michael Pinelis and David Ruppert of US’ Cornell University used macroeconomic data to forecast the monthly prevailing volatility in the US stock market, in addition to the expected return. Previous studies have focused on just the monthly expected return, or predicted both components with simple linear models.
The authors explain: “Reward-risk timing is the combination of both return- and volatility-timing. Return-timing can be profitable with superior forecasting ability, yet ignoring the risk associated with a high return, for instance, would lead to poor risk-adjusted performance. Timing the market with both the expected return and volatility addresses the drawbacks of these individual approaches.”
According to the authors, machine learning helps to provide more accurate estimates by taking advantage of complex non-linear relationships between market variables to help make optimal decisions. The machine learning model they used, Random Forest, grows multiple uncorrelated decision trees and outperforms linear methods due to its allowance of non-linear predictor interactions. The forecast of a decision tree is the mean of the observations that match the current observation’s criteria. The Random Forest forecast is the average of all the individual decision tree forecasts, stabilising the predictive performance.
The weight of the equity index in their portfolios rebalanced monthly is proportional to the expected excess return and inversely proportional to the squared volatility estimate. The authors note: “Reward-risk timing in this way offers economically substantial improvements in risk-adjusted returns, specifically, a 28% increase in Sharpe ratio.” They conclude: “The empirical results overall indicate a significant advantage in using machine learning for portfolio allocation.”
###
Contact the corresponding author: Michael Pinelis, mdp93@cornell.edu
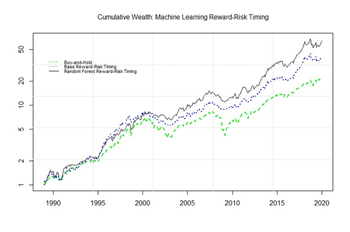